The Bias Problem in AI: Addressing Fairness and Diversity
September 15th, 2023
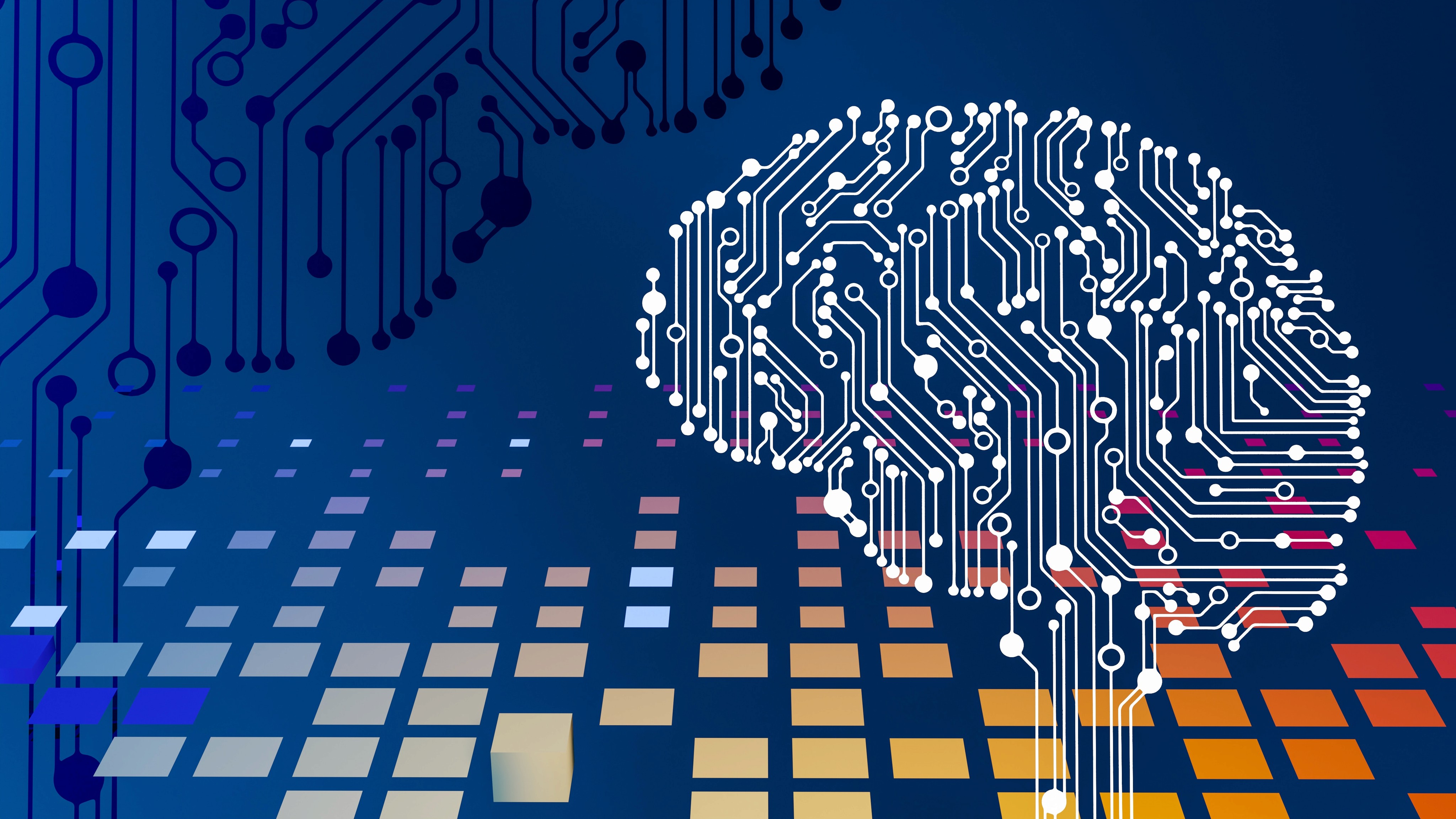
The Bias Problem in AI: Addressing Fairness and Diversity
September 15th, 2023
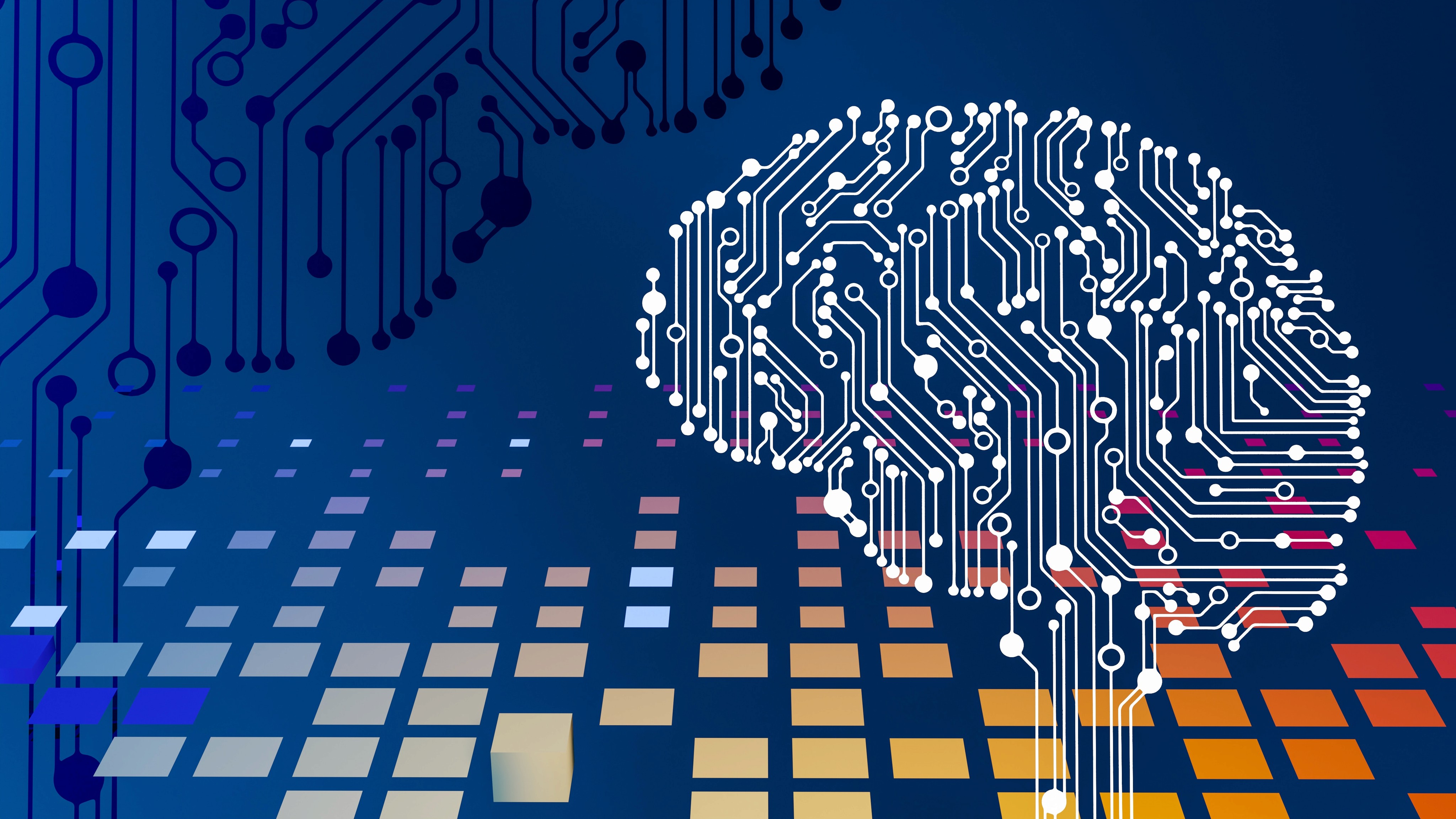
The Bias Problem in AI: Addressing Fairness and Diversity
September 15th, 2023
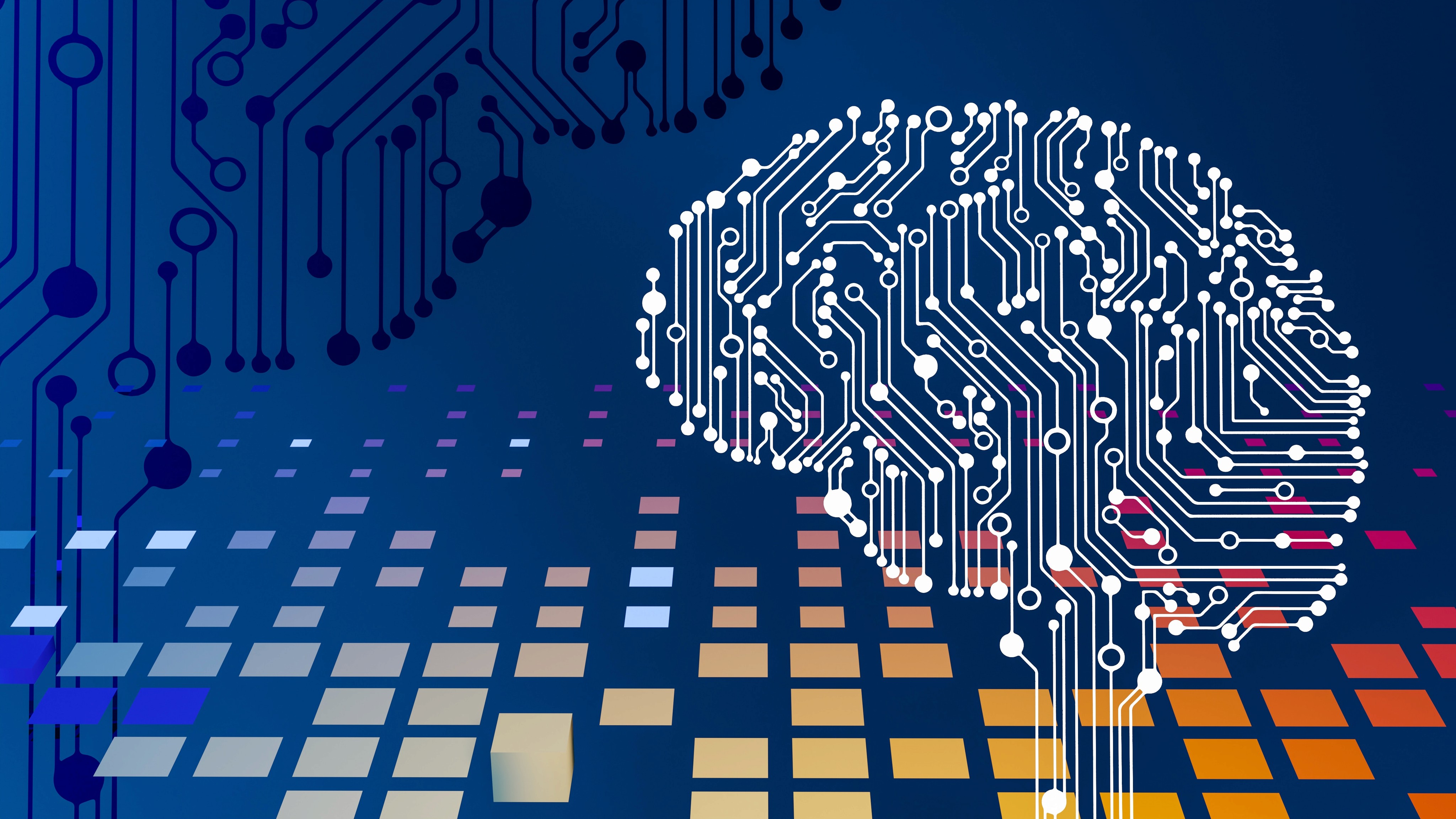
Understanding AI Bias
AI systems learn from data, and if the training data is biased, the AI model will inherit those biases. Bias in AI can manifest in various forms, including gender, race, ethnicity, and socioeconomic status. For example, biased hiring algorithms may favor one group over another, or recommendation systems may reinforce stereotypes. The consequences of biased AI can be far-reaching, from reinforcing inequality to perpetuating social injustices.
Challenges in Identifying and Mitigating Bias
One of the significant challenges in addressing bias in AI is the opacity of some machine learning models. Complex models, such as deep neural networks, can be challenging to interpret, making it difficult to identify and understand the sources of bias. Additionally, bias can be subtle and emerge in unexpected ways, making it a nuanced problem that requires careful examination.
Strategies to Address Bias in AI
Diverse and Inclusive Data: One of the fundamental steps in mitigating bias is to ensure that training data is diverse and representative. This means collecting data from a wide range of sources and ensuring that it includes underrepresented groups.
Bias Detection Tools: AI developers can employ specialized tools that help identify and measure bias in their models. These tools can flag potential bias issues, allowing developers to make informed adjustments.
Explainable AI (XAI): XAI techniques aim to make AI models more transparent and interpretable. By understanding how a model makes decisions, it becomes easier to identify and rectify biased patterns.
Ethical AI Guidelines: Organizations should establish clear ethical guidelines for AI development, outlining principles of fairness, transparency, and accountability. These guidelines can serve as a foundation for responsible AI practices.
Bias Mitigation Algorithms: AI researchers are developing algorithms specifically designed to reduce bias in AI systems. These algorithms can help mitigate the impact of biased data during training.
Conclusion: A Commitment to Fair and Ethical AI
The bias problem in AI is a complex and multifaceted challenge, but it is one that the AI community is actively addressing. To ensure that AI benefits all of humanity, we must commit to fairness, diversity, and equity in AI development. By employing diverse data, transparent models, and ethical guidelines, we can work toward a future where AI systems promote equality, rather than perpetuating bias and discrimination. In this way, we can harness the power of AI to build a more inclusive and just world for all.
Understanding AI Bias
AI systems learn from data, and if the training data is biased, the AI model will inherit those biases. Bias in AI can manifest in various forms, including gender, race, ethnicity, and socioeconomic status. For example, biased hiring algorithms may favor one group over another, or recommendation systems may reinforce stereotypes. The consequences of biased AI can be far-reaching, from reinforcing inequality to perpetuating social injustices.
Challenges in Identifying and Mitigating Bias
One of the significant challenges in addressing bias in AI is the opacity of some machine learning models. Complex models, such as deep neural networks, can be challenging to interpret, making it difficult to identify and understand the sources of bias. Additionally, bias can be subtle and emerge in unexpected ways, making it a nuanced problem that requires careful examination.
Strategies to Address Bias in AI
Diverse and Inclusive Data: One of the fundamental steps in mitigating bias is to ensure that training data is diverse and representative. This means collecting data from a wide range of sources and ensuring that it includes underrepresented groups.
Bias Detection Tools: AI developers can employ specialized tools that help identify and measure bias in their models. These tools can flag potential bias issues, allowing developers to make informed adjustments.
Explainable AI (XAI): XAI techniques aim to make AI models more transparent and interpretable. By understanding how a model makes decisions, it becomes easier to identify and rectify biased patterns.
Ethical AI Guidelines: Organizations should establish clear ethical guidelines for AI development, outlining principles of fairness, transparency, and accountability. These guidelines can serve as a foundation for responsible AI practices.
Bias Mitigation Algorithms: AI researchers are developing algorithms specifically designed to reduce bias in AI systems. These algorithms can help mitigate the impact of biased data during training.
Conclusion: A Commitment to Fair and Ethical AI
The bias problem in AI is a complex and multifaceted challenge, but it is one that the AI community is actively addressing. To ensure that AI benefits all of humanity, we must commit to fairness, diversity, and equity in AI development. By employing diverse data, transparent models, and ethical guidelines, we can work toward a future where AI systems promote equality, rather than perpetuating bias and discrimination. In this way, we can harness the power of AI to build a more inclusive and just world for all.
Understanding AI Bias
AI systems learn from data, and if the training data is biased, the AI model will inherit those biases. Bias in AI can manifest in various forms, including gender, race, ethnicity, and socioeconomic status. For example, biased hiring algorithms may favor one group over another, or recommendation systems may reinforce stereotypes. The consequences of biased AI can be far-reaching, from reinforcing inequality to perpetuating social injustices.
Challenges in Identifying and Mitigating Bias
One of the significant challenges in addressing bias in AI is the opacity of some machine learning models. Complex models, such as deep neural networks, can be challenging to interpret, making it difficult to identify and understand the sources of bias. Additionally, bias can be subtle and emerge in unexpected ways, making it a nuanced problem that requires careful examination.
Strategies to Address Bias in AI
Diverse and Inclusive Data: One of the fundamental steps in mitigating bias is to ensure that training data is diverse and representative. This means collecting data from a wide range of sources and ensuring that it includes underrepresented groups.
Bias Detection Tools: AI developers can employ specialized tools that help identify and measure bias in their models. These tools can flag potential bias issues, allowing developers to make informed adjustments.
Explainable AI (XAI): XAI techniques aim to make AI models more transparent and interpretable. By understanding how a model makes decisions, it becomes easier to identify and rectify biased patterns.
Ethical AI Guidelines: Organizations should establish clear ethical guidelines for AI development, outlining principles of fairness, transparency, and accountability. These guidelines can serve as a foundation for responsible AI practices.
Bias Mitigation Algorithms: AI researchers are developing algorithms specifically designed to reduce bias in AI systems. These algorithms can help mitigate the impact of biased data during training.
Conclusion: A Commitment to Fair and Ethical AI
The bias problem in AI is a complex and multifaceted challenge, but it is one that the AI community is actively addressing. To ensure that AI benefits all of humanity, we must commit to fairness, diversity, and equity in AI development. By employing diverse data, transparent models, and ethical guidelines, we can work toward a future where AI systems promote equality, rather than perpetuating bias and discrimination. In this way, we can harness the power of AI to build a more inclusive and just world for all.